Artificial Intelligence and Machine Learning: The sensXPERT Way of AI
The plastics industry is one of the many industries currently being transformed by artificial intelligence (AI). Inspired by human cognitive behavior, this revolutionary technology enables machines to perform tasks such as recognizing speech, images, and patterns. The potential applications of modern AI and machine learning (ML) are expansive. Still, in the plastics industry, their use can result in rewarding developments such as optimized production processes, cost reduction, and improved part quality.
There is often some confusion around the terms AI and machine learning, so in this post, we aim to explain what they are, clarify the difference between the two, introduce various approaches currently in use, and highlight the applicability of AI and ML in the plastics industry.
This blog post is part of a series on the sensXPERT way of AI. The first two articles introduce AI, machine learning, and data science and position them in the plastics manufacturing industry. The final article delves into sensXPERT’s use of AI to enhance production processes, transparency, and quality control.
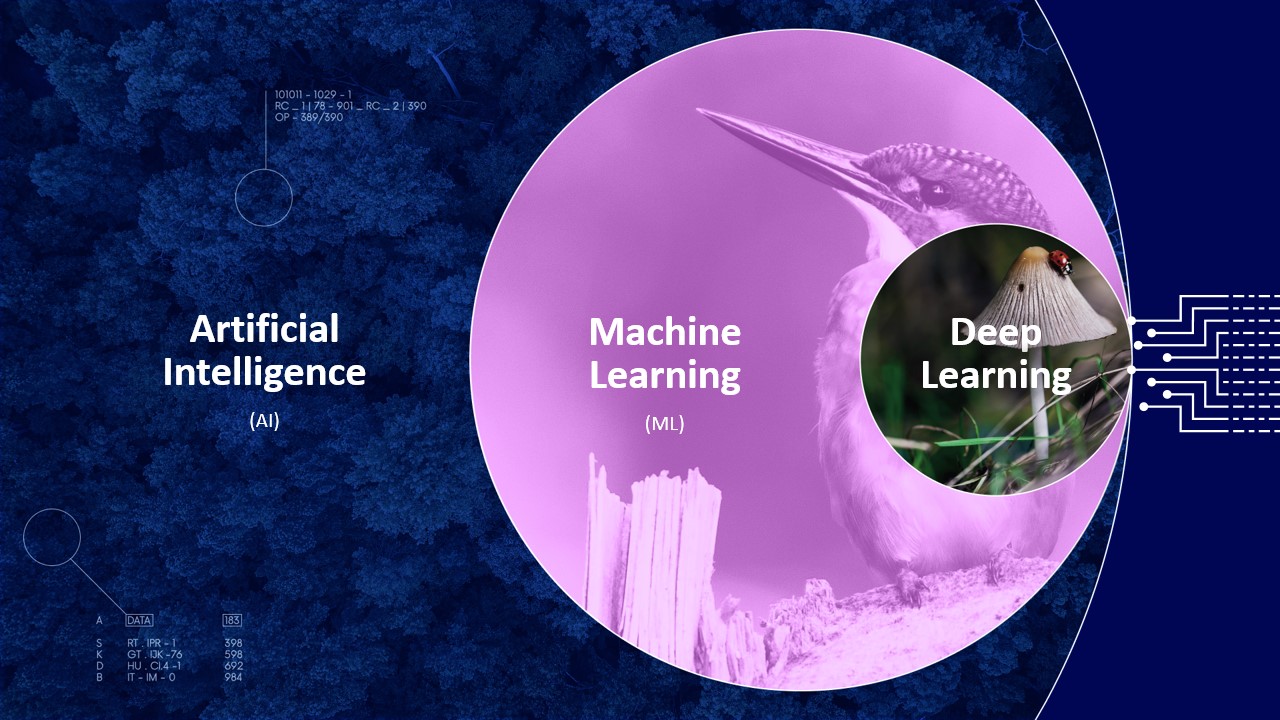
What is artificial intelligence?
Although the concept has been prevalent in myth and storytelling for centuries, the modern-day notion of “artificial intelligence” was first introduced in the 1950s as a result of significant progress made in the fields of neurobiology, mathematics and information theory research. Since the 1950s, AI has developed tremendously and has witnessed a vast amount of applications and advancements. So what exactly is AI?
Simply put, artificial intelligence – a subsection of cognitive science – is the simulation of human cognitive behavior in machines. On account of AI, machines now have the capability of performing various, mundane tasks that were once the exclusive domain of humans. Additionally, AI is also able to solve tasks that might prove more complex for humans, such as clustering large dimensional data or car design optimization. Artificial intelligence can be attained by using predefined decsion trees, as well as fixed behavior coding.
Being a “future key technology”, the development of AI is likely considerably economically driven [1]. Nevertheless, the rapid acceleration of AI development in recent years can be predominantly attributed to advances in computing power, the availability of large datasets, and breakthroughs in machine learning and deep learning algorithms.
What is machine learning?
As a subset of AI, machine learning enables machines to learn from data and develop their intelligence. Consequently, the machines do not need to be explicitly programmed to perform specific tasks. In ML, algorithms are used to analyze and discern patterns from data. Machines are then trained to wield these patterns and make decisions or predictions. Following ML training, machines are able to apply their gained knowledge and generate predictions out of new data sets.
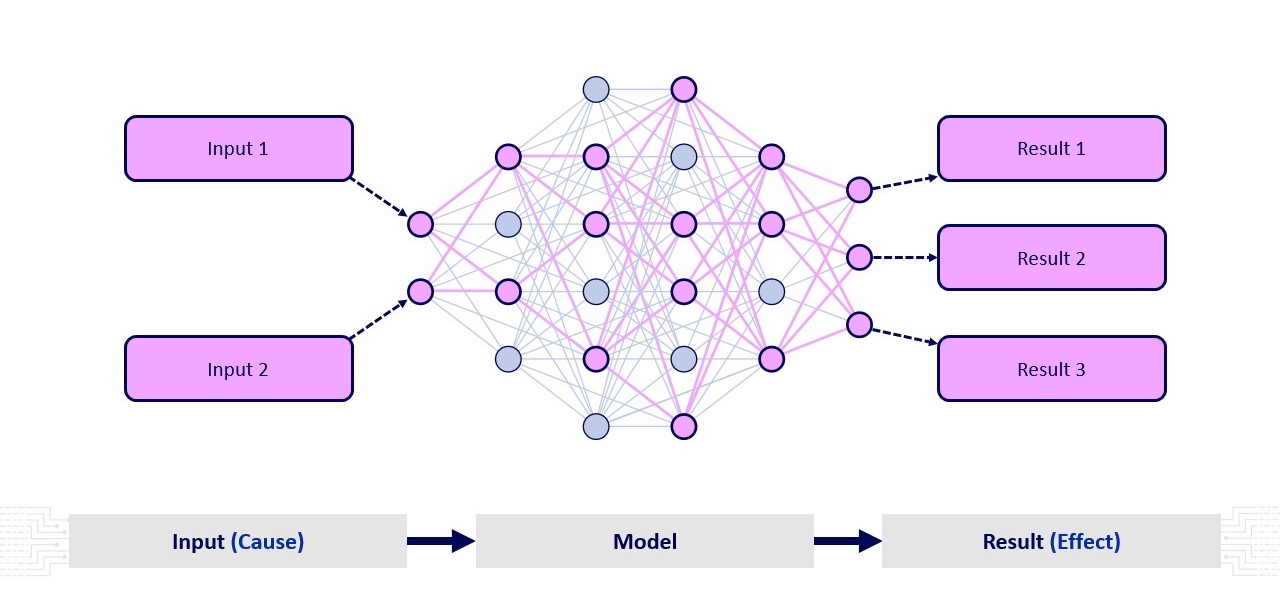
A considerable advantage of machine learning is its capacity to contend with expansive data. On account of the massive surge in data as of late, ML has been a powerful tool in data set processing and analysis, the extraction of valuable insights, and data-driven decision-making.
Approaches in machine learning
When it comes to applying machine learning, there are three most commonly used approaches, each with advantages depending on their application.
1. Supervised Learning
In supervised learning, labeled data sets are presented to the ML algorithm. A “supervisor” informs the machine of the correct outputs or desired target variables. Accordingly, the machine is trained to deduce patterns from and learn the relationship between the input data and the target output. During training, the machine receives feedback on its answers and predictions from a supervisor to ensure validity.
Based on the machine’s acquired learnings, it can make accurate predictions about new, unfamiliar data. Common supervised ML tasks include good/bad part classification and regression. Examples of supervised learning applications include human pose detection and, more generally, object detection.
2. Unsupervised Learning
In unsupervised learning, data sets without labels, or target variables, are presented to the ML algorithm. The machine is then tasked with making inferences and finding structure in the vast data. Unlike supervised learning, the machine receives no feedback in unsupervised learning, and is therefore responsible for discovering meaningful patterns on its own.
This method is frequently used for clustering, dimensionality reduction, and anomaly detection. One example of its application is recommendation systems, which are used in many industries to provide users with suggestions based on their interests.
3. Reinforcement Learning
Reinforcement learning is an approach through which the machine, or agent, learns by trial and error. In this case, there is no supervisor involved. However, the feedback-heavy approach affirms the agent’s appropriate responses by being rewarded after having reached a desired goal. Correspondingly, the agent receives “penalties” should its response be unsuitable.
Unlike in supervised learning, there is no feedback provided on whether an action is “correct” or not in this approach. The algorithm must independently identify suitable strategies by exploring and refining different approaches.
Reinforcement learning aims to teach an agent to seek optimal results and accumulate as many rewards as possible. This ML technique has been used to develop autonomous agents like self-driving cars.
Before delving into machine learning in the plastics manufacturing industry, one ML method is worth looking into.
What is deep learning?
Often confused with one another, deep learning is a branch of machine learning rather than a synonym for it. Deep learning uses neural networks with multiple layers to create models and solve complex problems or tasks. What makes it “deep” learning are the various layers that make up the neural networks and expand their capacity to handle complex computations and generate accurate outcomes. In other, previously popular ML methods, features in the data used to be detected manually. Deep learning is advantageous in that it learns to detect features in the data itself.
Generally, deep learning models call for incredible amounts of data, unlike more traditional machine learning models, such as random forest or support vector machine. Not only does this make deep learning more expensive, but it is also very challenging to train and apply. At the same time, this ML subfield is highly popular for its ability to address and solve some of the most elaborate issues in various industries; plastics manufacturing is one of them.
Applying ML in the Plastics Industry
The plastics industry is expansive and strongly relies on process stability and simulation, material characterization and modeling, and a skilled workforce. Understanding different process parameters, deviations, and improvement possibilities is imperative to ensure high-quality production. With several production stages, from supplying raw materials and manufacturing parts to distribution and retail, efficiency and sustainability are all-encompassing goals.
That is where machine learning comes into play. ML algorithms can be used in robotics for industrial automation. Additionally, in process control, ML can provide real-time insight into material behavior and process trajectories. A combination of material science and ML algorithms can play a significant role in optimizing manufacturing processes.
Adopting artificial intelligence in manufacturing can be challenging, as the models need to be customized for specific machines, materials, and environments. However, applying these models is utterly possible, especially when collaborating with AI engineers, subject matter experts, and data scientists.
In our next post, we’ll delve deeper into data science’s role in artificial intelligence and machine learning.
Sources:
[1] Seifert, I. et al., (2018, July). Potential of Artificial Intelligence in Germany’s Producing Sector. PAiCE Scientific Assistance.