Feedback Loops, Model Validation, and Retraining: sensXPERT’s Accuracy
sensXPERT Digital Mold is a process control solution that empowers manufacturers to optimize and digitize their plastics manufacturing processes. Predictive machine learning models are central to this technology, as they determine the exact point at which processed materials will have reached a certain degree of cure or crystallization. Therefore, Digital Mold relies heavily on the accuracy and effectiveness of the models it wields.
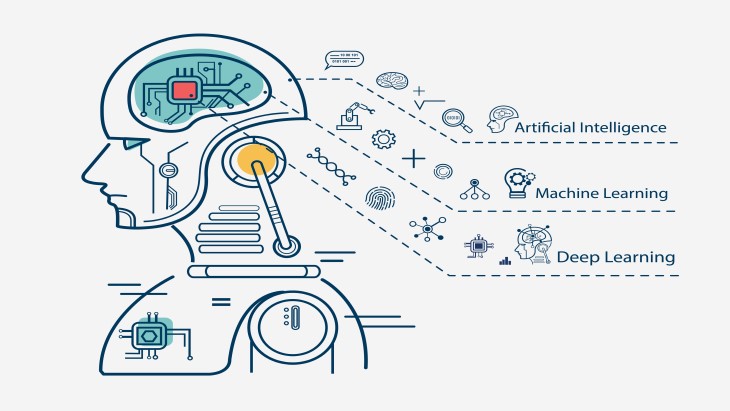
How does sensXPERT ensure that our models maintain reliability and precision, and how can customers be assured that their newly predictive process will generate products of outstanding quality? The answer is a combination of feedback loops, model validation, and retraining.
In this article, we will explain how models are trained and validated, discuss the importance of model validation and retraining, as well as take a look at predictive analytics in manufacturing. We will then clarify the ways by which the sensXPERT team guarantees the accuracy of the Digital Mold machine learning models.
Training and Validating Machine Learning Models
In an article on artificial intelligence and machine learning, we defined machine learning as “a subset of AI, machine learning enables machines to learn from data”. Machine learning models aim to resolve a problem by analyzing patterns and trends in certain data sets. These patterns are subsequently used to generate decisions or predictions.
The entire machine learning process begins with a dataset. As a means of avoiding biases in the resulting model’s decision-making process, it is essential to split the data set. Dataset splitting is the process of dividing a dataset into two or more subsets for model training, testing, and possibly validation.
Dataset splitting ensures the reliability of the model, its ability to generalize to new data, and its effectiveness in real-world scenarios. There are various types of data splits, such as the train-test split in which the test data is held out during training.
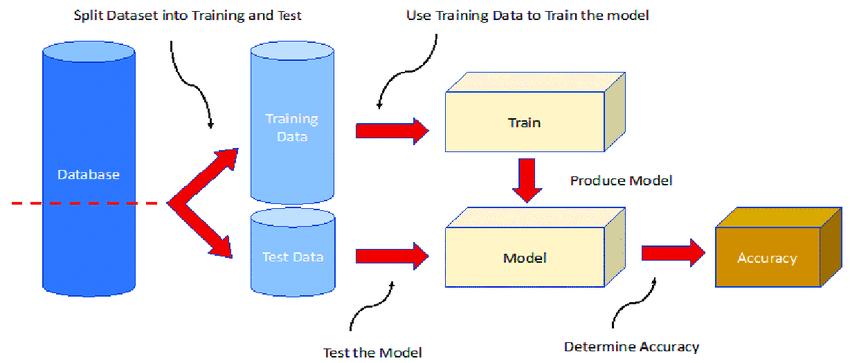
Source: https://www.researchgate.net/figure/Training-and-validation-scheme-for-machine-learning-methods-The-database-is-split-and_fig1_357570421
The training set is used to train the machine learning model, and the testing set is used to validate its performance. Typical split ratios are 70:30 and 80:20, so that the majority of the dataset is used to train the model.
The Importance Model Validation and Retraining
After machine learning models are trained, the resulting model is validated against a testing dataset to assess its performance on new, unseen data and to make sure it is prepared for deployment in a real-world scenario. Model validation is an important step in the model development process because it guarantees the accuracy of the model’s outputs.
If there are multiple models to develop, the training is conducted on the test set and the model selected is that which performs the best. In this case, a third data set is required to validate the model on unseen data.
Once a model has been deployed, retraining is a crucial step in reinforcing a model’s accuracy. Machine learning retraining is the process of updating and improving the trained model using new data. Frequent retraining leads to the continued relevance, accuracy, and adaptability of a model when faced with changing circumstances; data drifts or parameter changes for example.
So, how are sensXPERT’s machine learning models used and refined in a plastics manufacturing environment?
Predictive Analytics in Plastics Manufacturing
As initially mentioned, sensXPERT uses machine learning models to predict the point at which a part in a plastics manufacturing production cycle will have reached a manufacturer’s desired degree of cure or crystallization.
Several setbacks can hinder plastics manufacturing processes – including quality fluctuations, material deviations, a lack of transparency, and, resultingly, final part defects – therefore, predictive analytics are gaining significant traction. Technologies and innovations that predict potential system failures, optimize production schedules, and forecast inventory demand, have been developed to help streamline plastics production and avoid unnecessary downtime.
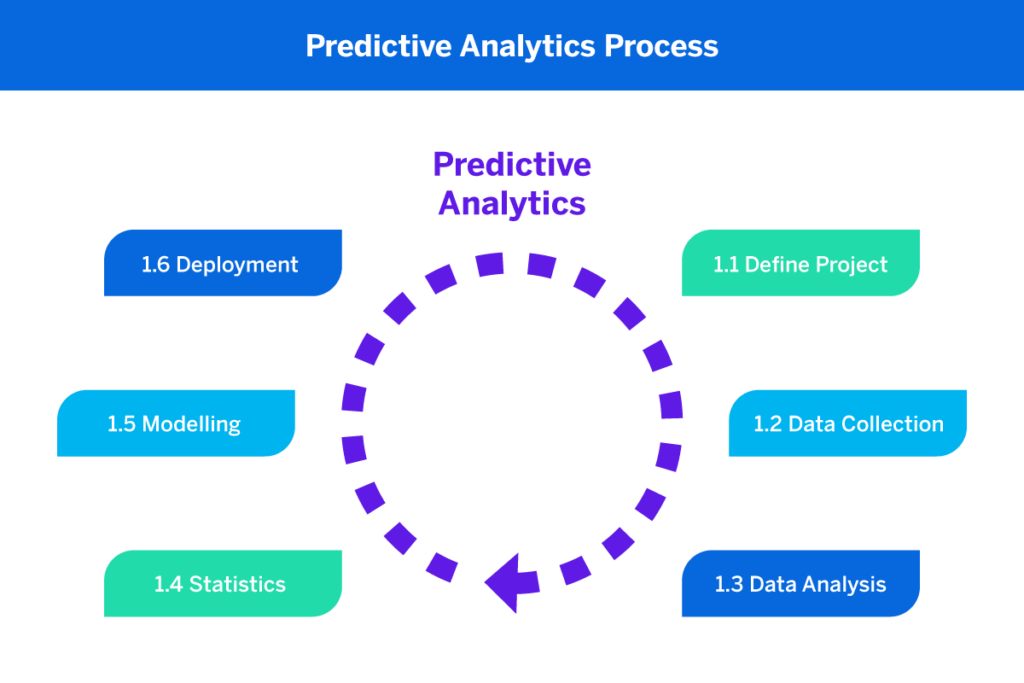
Source: What is Predictive Analytics? | Qualtrics
sensXPERT uses predictive analytics to look directly into molding machines and forecast the outcomes of running production cycles to enable dynamic process control. sensXPERT Digital Mold allows manufacturers to perform quality control on every part produced, remove their safety buffers, reduce cycle times, benefit from less scrap, and increase their overall cost-efficiency.
At the heart of this solution lies sensXPERT’s machine learning models. The models are created based on process datasets – as elaborated upon in previous sections – and strong feedback loops. For instance, a customer will provide the sensXPERT data science team with several sets of process data. The customer will also clarify which cycles were acceptable and which cycles were problematic, alongside more specific process information. Problematic cycles may have contamination, short fill, warpage, ageing, or other similarly suboptimal characteristics.
Using this feedback, the sensXPERT team can adequately train a model using the holdout method described above. Once the model has been trained and deployed, the sensXPERT team conducts systemic validation experiments on the model to ensure its continued accuracy.
Relying Feedback Loops
Feedback loops are pivotal in sensXPERT’s maintenance of machine learning models. Should a customer alter their process parameters, for example, relaying prompt feedback to sensXPERT allows the team to validate and ensure that the model accurately conveys how certain changes and fluctuations affect process outcomes. Therefore, the model can effectively contribute to successful process control and optimization.
In ‘Presenting: The sensXPERT Way of AI’ we describe how we use machine learning models to enable polymer process optimization. In summary, the sensXPERT Edge Device hosts machine learning models and deploys them on real-time data collected by sensXPERT’s in-mold material characterization sensors to generate a prediction on the most optimal point at which to end a cycle.