Presenting: The sensXPERT Way of AI
We’ve covered artificial intelligence (AI), machine learning (ML), data science, and several other relevant approaches in our previous articles. With applications in a myriad of industries, every new development in the realm of AI, ML, and data science inspires the advancement of new technologies and possibilities for improvement.
So now, in our final article on the sensXPERT way of AI, we would like to bring you along as we take a closer look at our technology and use of AI to address challenges in the plastics manufacturing industry.
Artificial Intelligence in the Plastics Industry
AI is making strides in the plastics industry. Whether by generating consumer demand predictions or spotting irregularities in machinery, AI can increase efficiency, reduce costs, conserve energy expenditure, and enable sustainable production. As will be demonstrated, the plastics industry is confronted with a number of challenges and setbacks, making the advancement of AI and machine learning a timely and advantageous one.
Several AI solutions are being developed to combat inefficiency, waste and to improve operations and sustainable practices in the plastics industry. Examples include solutions designed to predict potential system failures, improve supply chain operations, simulate sustainable package designs, and detect environmentally friendly plastic disposal.
When it comes to plastic production, more specific challenges hamper manufacturing processes.
Processing Challenges and Setbacks
Several issues related to process transparency, stability, and quality assurance disadvantage plastics processors. Such challenges generally result in rising energy and material costs. Additionally, the introduction of new materials, such as bio and reusable plastic, is another challenge on established processes. Therefore, emerging technologies are essential to combat the concerns and difficulties in the industry.
Detecting defects and their causes
A manufacturing process typically consists of quality control prior to and after a part is produced. Unfortunately, a lack of transparency during processing impacts quality assurance engineers’ ability to pinpoint the origin and cause of part defects. Additionally, several other defective parts could have been produced in the time it takes engineers to detect substandard parts. Thus, limited transparency can lead to material waste and redundant energy consumption.
Quality fluctuations and material deviations
Correspondingly, material behavior can be inconsistent and fluctuate due to any number of influences. Factors such as production batch, temperature, transfer, storage, aging, and more influence material behavior. In line with the previous challenge, deviations and quality fluctuations are difficult to account for without in-process transparency.
The manufacturing ‘black box’
The challenges above are troublesome and inconvenient owing to the manufacturing ‘black box.’ Processors have insight into the material before the manufacturing process and post-process insight into the part produced. However, everything between those two stages can be considered a ‘black box’ of sorts. A real-time understanding of material behavior and in-mold conditions during processing is lacking in the industry.
Fortunately, new technologies with AI capabilities are on the rise and able to address the highlighted challenges.
sensXPERT Digital Mold
The sensXPERT Digital Mold is one such technology, which you can read more about in this article.
“For the majority of companies in plastics processing, the entry into and benefit from AI is not obvious. At sensXPERT, we combine material science and process data via machine learning algorithms and continuously increase their scope through deep learning. The multitude of parameters in manufacturing overwhelm our human way of thinking. Here, AI shows its potential, visualizes hidden interrelations and presents added value in a very short time.”
– Dr. Alexander Chaloupka
The sensXPERT process control solution by NETZSCH Process Intelligence GmbH aims to supply plastics processors with real-time process data and material characterization to determine ongoing conditions within molds and predict process outcomes. In doing so, processors can perform quality control on each part produced, decrease cycle times, and benefit from lower costs, less material waste, and overall sustainable production.
A significant part of the sensXPERT Digital Mold lies in its machine learning capabilities.
A look at our machine learning magic
As you might remember from our first article of the series, supervised learning is a machine learning approach. A “supervisor” provides the desired outputs and continuously offers feedback to a machine, which learns to derive patterns and relationships from the data. At sensXPERT we use the supervised learning approach to train our ML models.
So, what does that entail?
The sensXPERT Digital Mold comprises ML-based technology that can predict production cycle outcomes using predictive algorithms. The solution consists of four main elements:
- In-mold sensors
- An accompanying edge device
- An edge device interface (Web App)
- The Digital Cloud Service
Regarding machine learning, the edge device hosts ML models and applies them to the real-time data collected by the device. The visualization of processes and application of ML models are presented on the Web App, so operators can view and adapt running cycles. Machine learning models require regular retraining to adapt to changing conditions. Therefore, the Cloud securely stores all incoming data for the purpose of retraining. By leveraging this stored data, we ensure that our models stay current and capable of providing accurate insights in dynamic environments. The image below demonstrates all aspects of the sensXPERT Digital Mold solution and their exchange with one another.
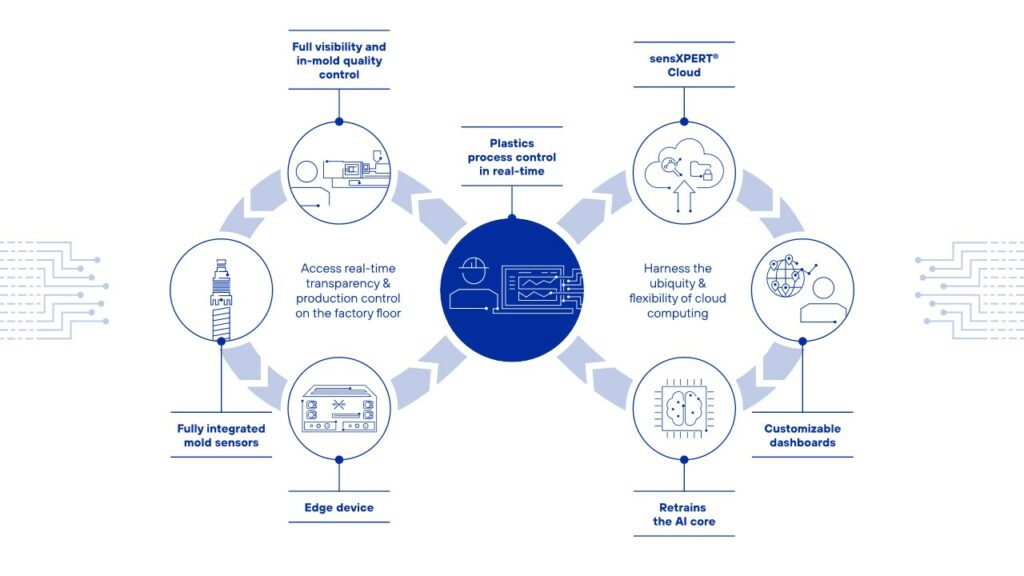
For sensXPERT to successfully forecast cycle outcomes, data is crucial to the solution. Therefore, consistent production using the Digital Mold product enables regular data collection. Data inputs are used to train the ML algorithm through supervised learning after collection. The inputs include sensor signals, in-mold temperature, and kinetic models.
What are kinetic models?
In the thermoset world, kinetic models are a way of mathematically describing a chemical reaction at specific temperatures. These models illustrate the speed of curing or vulcanization in thermosets and elastomers, respectively. As a means of predicting process outcomes, sensXPERT determines optimal points of cure (Degree of Cure, DoC) using kinetic models.
Subsequently, the ML algorithm outputs DoC and glass transition temperatures, which play a role in detecting variations in process and material behavior. The algorithm compares data patterns in running cycles with patterns learned from historical data. ML algorithms consider any potential deviations within a cycle and predict process outlooks accordingly. To put it simply, machine learning at sensXPERT involves data collection, algorithm training via supervised learning, model generation, and, finally, cycle outcome predictions.
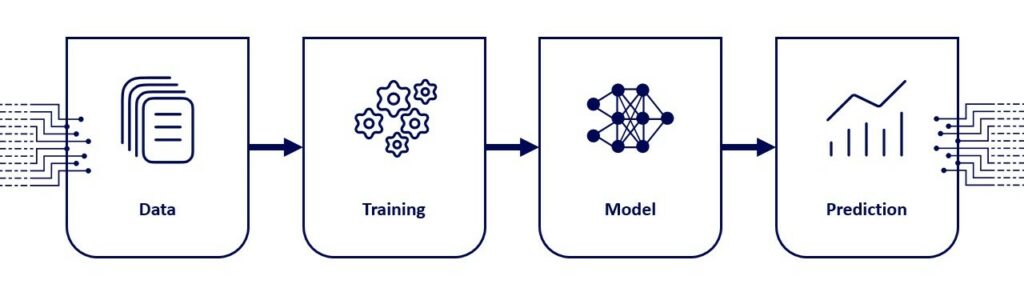
The illustration below is an example of one such prediction generated using machine learning models. The pink line represents temperature, the dark blue line signifies a predefined target value for DoC, the light blue dashed line presents measured data, and the green dashed line depicts the predicted cycle outcome.
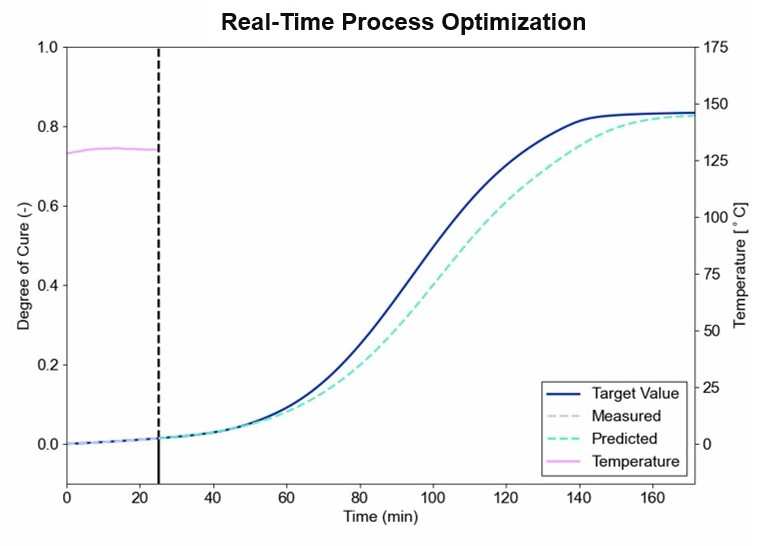
sensXPERT & AI: From Difficulties to Dynamic Processes
We started this post by outlining several difficulties that impact plastic processors. In summary, the challenges we mentioned were detecting defects and their causes, facing quality fluctuations and deviations in material behavior, and the manufacturing ‘black box.’ sensXPERT combats each one of these issues with the Digital Mold solution. Our solution’s ML algorithms ensure that manufacturers and process operators will ultimately gain transparency, avoid part defects, and implement dynamic process adjustment.
Predictive algorithms and visualizing them through the Web App give operators greater control over process outcomes. No longer is there difficulty in detecting defects and pinpointing their origins. No longer are there final part quality fluctuations with the Digital Mold solution accounting for material behavior deviations. No longer will the manufacturing’ black box’ be a problem. sensXPERT creates total process transparency and uses machine learning to address industry challenges.
With that, we have come to the end of our series on the sensXPERT way of AI but stay on the lookout for new posts! If you would like to be informed of new sensXPERT content, subscribe to our newsletter and be the first to catch the latest industry insights.
You can check out the previous sensXPERT way of AI articles here: