Detecting Material Aging in Automotive Composite Production
Key Facts

With constant pressure to increase production efficiency, the automotive industry cannot afford to have improper storage conditions and material aging, which can lead to reduced material workability, increased scrap rates, and disruptions in production flow.
To prevent these negative aging effects, a leader in the automotive industry collaborated with sensXPERT to detect material aging in their processing of carbon fiber reinforced polymers.
sensXPERT’s analysis resulted in successful regression model training, thus setting the stage for in-mold anomaly detection. The combination of advanced modeling and real-time process monitoring holds significant potential for streamlining production and maximizing output.
Central to this success story is a leader in the automotive manufacturing landscape who boasts a robust network of production facilities, allowing them to deliver a diverse range of vehicles to meet market demands.
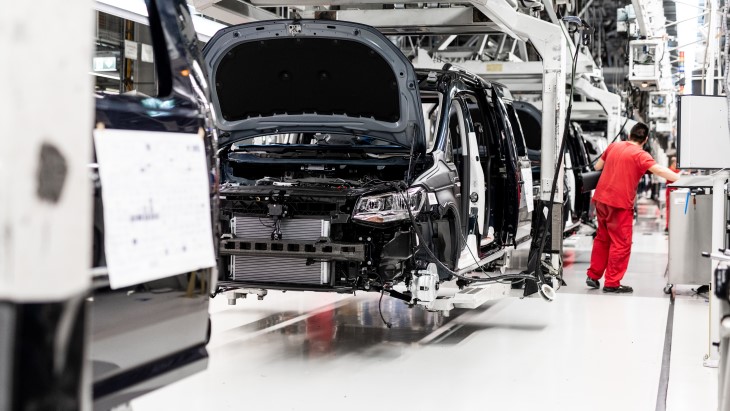
The automotive industry experiences constant pressure to increase production efficiency, while dealing with evolving regulations, rapidly developing technologies, and a growing demand for lighter, more fuel-efficient vehicles.
Within this demanding environment, efficiently managing raw material storage is a critical factor for this industry leader. Their extensive production network requires a constant flow of diverse materials, and even slight inefficiencies in storage conditions can have a domino effect on overall production efficiency. Improper storage conditions can accelerate material aging, leading to:
- Reduced Material Workability: Aged materials may become brittle, less pliable, or more susceptible to defects during manufacturing processes. This can significantly slow down assembly lines, requiring additional work which eats into production time and resources.
- Increased Scrap Rates: Materials that have been improperly stored or that present aging issues can become scrap, resulting in wasted resources and additional costs. This is especially concerning when using expensive raw materials like advanced composites used in cutting-edge vehicle technologies.
- Disruptions in Production Flow: Unexpected material shortages due to unforeseen aging issues can cause significant disruptions in production schedules.
To prevent the negative effects of material aging, the automotive leader approached sensXPERT with the initial samples of data collected from a series of measurements that they conducted to see whether they could detect material aging in their processes.
Laboratory Test Details
The automotive OEM conducted 136 measurements on an epoxy CF-SMC material. In order to investigate aging effects on the material, different samples were stored at three different ambient temperatures for up to 70 days. The three ambient temperatures were room temperature, 0°C, and -25°C.
After exposing the epoxy CF-SMC to various temperatures and storage times, the company cut out samples, placed them in a press with a processing temperature of 130°C, and used dielectric analysis (NETZSCH DEA288) to examine their curing behavior.
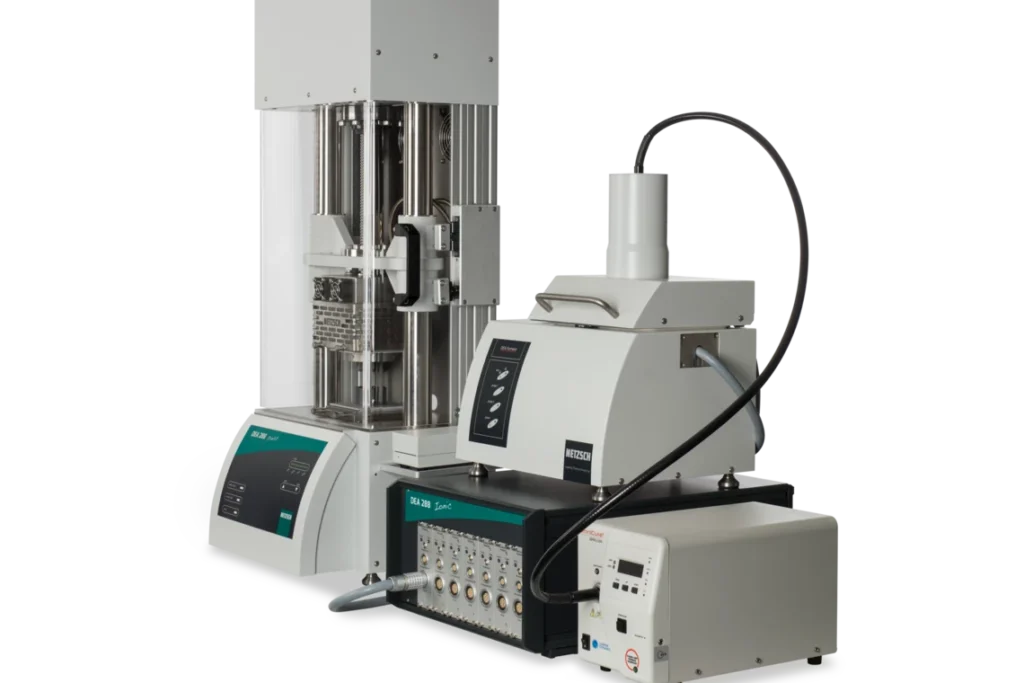
However, when evaluating the change in curing behavior of the different samples, the automotive company did not detect any systematic trends.
For this reason, the automotive leader sought out sensXPERT to uncover whether it would be possible to detect aging and the effects of aging on their materials based on their measurements.
sensXPERT Analysis
After receiving the automotive company’s measurements, the sensXPERT team divided the measurements according to the three different storage temperatures. This left the team with only about 40 measurements per storage temperature. With this being a limited amount of data, our team then clustered the data into four groups with samples showing similar curing behavior. More specifically, the data was clustered according to the number of storage days; 0-7 days, 14-28 days, 35-42 days, and 56-70 days.
Furthermore, the sensXPERT team visualized the 95 % confidence interval of each individual storage group to detect deviations in the material’s curing behavior based on the three different storage temperatures. The confidence intervals eliminate some spread while preserving the characteristic shape for each group.
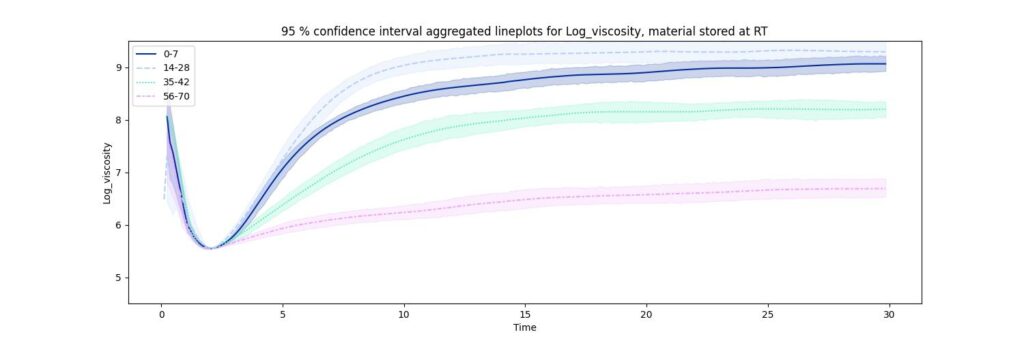
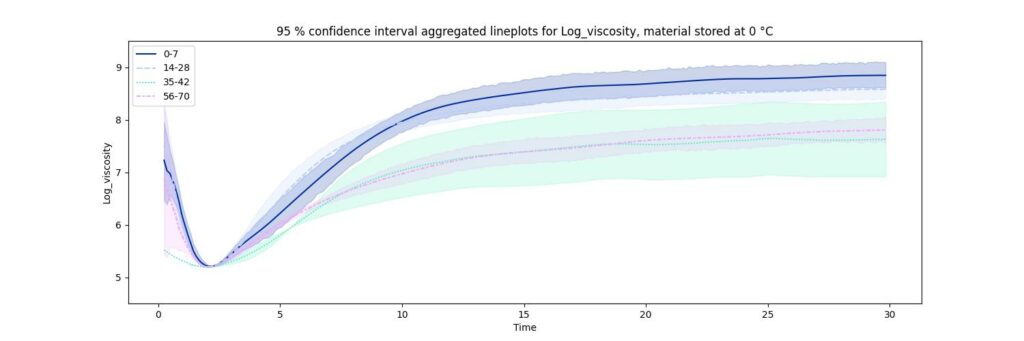
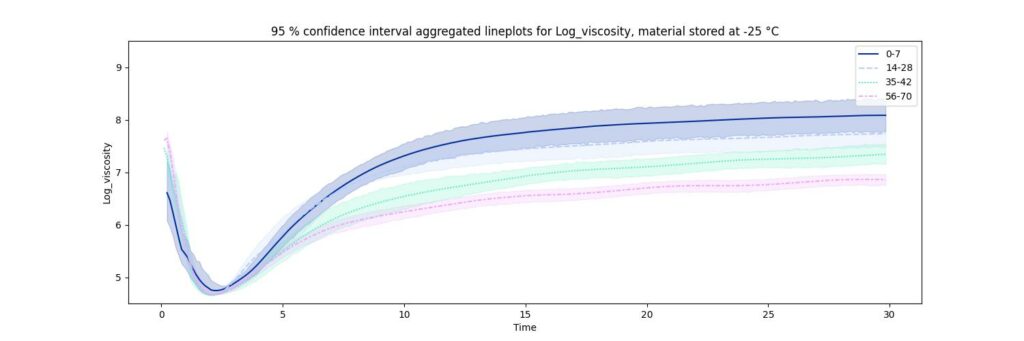
According to the log ion viscosity graphs, the curing behavior of the material that was stored at room temperature greatly differs per number of storage days, as is made visible in figure 1. However, the slope of the curves of the material stored at the other two temperature profiles – figures 2 and 3 – decrease less sharply in comparison to the room temperature curves.
One reason for these results could be that some resins exhibit ambient curing, meaning that the crosslinking reaction between the molecules begins at room temperature. While slow under these conditions, the formation for the 3D network characteristic of the resin begins even at room temperature. This initial stage creates a state of pre-crosslinking, where some crosslinks form, but the reaction is not complete.
As pre-crosslinking progresses, the material may reach a vitrified state. In this glassy state, the developing network becomes rigid, hindering the mobility of the remaining reactive groups. This limited mobility can become problematic during final cure, especially if the material is stored for extended periods.
Since the remaining reactive groups struggle to find each other due to pre-crosslinking and vitrification, they may not fully participate in the intended curing process. This leads to incomplete curing and compromises mechanical properties in the final product.
If ambient curing took place in this case, it is made visible by the pink curve in figure 1 – material stored at room temperature between 56 and 70 days – which presents almost no chemical reaction during curing. Additionally, the material stored under -25°C and 0°C had less deviations than that at room temperature
Also, figure 4 summarizes the analysis of the ionic viscosity slope. It presents the development of the maximum of the derivative per curve which depicts the most prominent characteristic of the material aging. Figure 4 illustrates that the characteristic decreases with a rising number of storage days but also that the decrease is less distinct with lower storage temperatures.
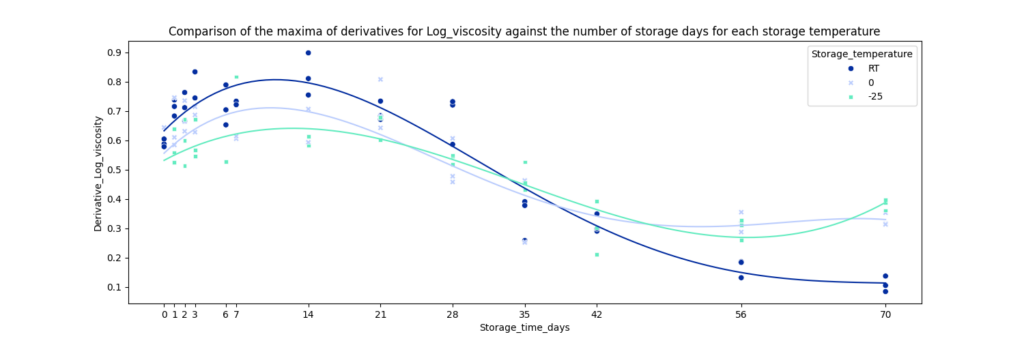
Beyond this initial analysis, the sensXPERT Team also trained and tested a machine learning model to be able to identify aging and eventually integrate the sensXPERT Digital Mold Solution into the customer’s production processes. In that case, the machine learning model would be able to detect material aging during processing, predict potential deviations accordingly, and dynamically adjust the process to ensure an optimal final part.
Model Training and Testing
To go about training and testing the machine learning model, the sensXPERT Data Science team extracted statistical features from the log ion viscosity curves. Then, the data was divided into a training and test set using a 70/30 split. As there was a limited amount of data available, the samples were also assigned into four groups based on storage days to train and test the model.
Figure 5 shows the confusion matrix for model inference on the test set. A confusion matrix opposes the true labels to the predicted labels, depicting the performance of the trained model. As is displayed in figure 5, the model was able to correctly predict each sample from the test set except for one misclassification where a sample belonging to the 0-7 group was predicted as 14-28.
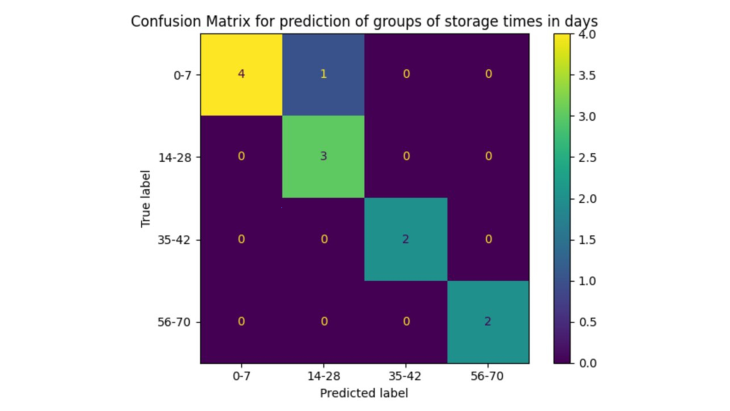
Finally, the model presented high accuracy in predicting the right category, regardless of the one misclassified sample. It should be noted that with a limited amount of data most machine learning models cannot generalize and that in this case more data is needed to make the model robust. With more samples per individual storing days, it is also possible to train a regression model that predicts the exact number of storage days instead of classifying a storage group.
Analysis Results
Based on the analysis and machine learning model outcomes, sensXPERT was able to come to several conclusions. First, the aging effects of the epoxy CF-SMC material used in the DEA tests present significant, non-linear changes in their behavior. Additionally, despite using a small data set, a machine learning model can accurately classify grouped storage times.
Looking ahead, by leveraging a larger data set, regression models can be trained, leading to more precise predictions and improved efficiency. When assessing the actual need for detecting materials that have been stored incorrectly or for too long, applying anomaly detection to this use case to detect any behavior that differs from an ideal curing process can be considered.
By identifying deviations from these learned optimal states, production teams can quickly react and address issues before they significantly impact efficiency or quality. Beyond production teams reacting, sensXPERT Digital Mold is also a solution that can dynamically adjust processes automatically through a stop trigger.
This combination of advanced modeling and real-time monitoring holds immense potential for streamlining production and maximizing output.